Cybersecurity in Data Analytics: Protecting Insights from Threats
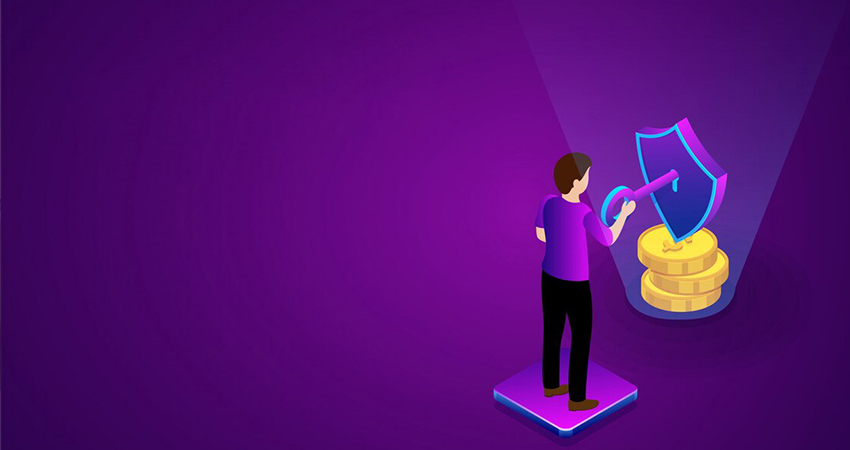
Data analytics plays a crucial role in modern decision-making, enabling organizations to extract valuable insights from vast amounts of data. However, with this reliance on data comes the growing risk of cybersecurity threats that can compromise data integrity, confidentiality, and availability. Protecting data analytics from cyber threats is essential to maintaining trust and ensuring business continuity.
Key Cybersecurity Threats in Data Analytics
- Data Breaches – Unauthorized access to sensitive data can lead to financial loss, reputational damage, and regulatory penalties.
- Malware and Ransomware – Malicious software can corrupt, encrypt, or steal critical data, disrupting analytics operations.
- Insider Threats – Employees or contractors with access to sensitive data may intentionally or unintentionally expose it to risks.
- Data Manipulation Attacks – Cybercriminals can alter datasets, leading to inaccurate analytics results and flawed decision-making.
- Cloud Security Risks – As organizations move their analytics operations to the cloud, weak access controls and misconfigurations can expose data to unauthorized users.
- AI and Machine Learning Vulnerabilities – Adversarial attacks on machine learning models can manipulate predictions and outcomes.
Best Practices for Securing Data Analytics
- Implement Strong Access Controls – Use multi-factor authentication (MFA) and role-based access control (RBAC) to restrict data access.
- Encrypt Data – Protect data at rest and in transit using advanced encryption protocols.
- Regular Security Audits – Conduct vulnerability assessments and penetration testing to identify potential weaknesses.
- Data Masking and Anonymization – Reduce exposure of sensitive data by masking or anonymizing personally identifiable information (PII).
- Secure Data Storage and Backup – Ensure redundant backups are stored in secure locations to prevent data loss from ransomware attacks.
- Monitor and Detect Anomalies – Deploy AI-powered cybersecurity tools to detect unusual access patterns and potential threats in real time.
- Educate Employees – Provide ongoing cybersecurity training to employees to recognize phishing attempts and follow best practices.
- Compliance with Regulations – Adhere to industry standards such as GDPR, HIPAA, and ISO 27001 to ensure legal and ethical data handling.
Future Trends in Cybersecurity for Data Analytics
- Zero Trust Architecture (ZTA) – Implementing a "never trust, always verify" approach to data access and security.
- Blockchain for Data Security – Utilizing blockchain to ensure data integrity and prevent unauthorized modifications.
- Automated Threat Intelligence – Leveraging AI-driven threat detection systems to enhance cybersecurity defenses.
- Quantum Cryptography – Developing quantum-resistant encryption techniques to protect against future cyber threats.
Conclusion
Cybersecurity in data analytics is not just a technical necessity but a strategic imperative for organizations that rely on data-driven decision-making. By adopting robust security measures, staying ahead of emerging threats, and fostering a culture of cybersecurity awareness, organizations can safeguard their valuable insights and maintain trust in their analytics systems.